Calculating Body Mass Index (BMI) in SPSS: A Guide
BMI, a measure of body fat, is widely used in medical research and healthcare. SPSS, a popular statistical software, offers tools for calculating BMI. Understanding how to calculate BMI in SPSS enables researchers to analyze data efficiently for various applications.
The historical development of BMI can be traced back to the 19th century, with Adolphe Quetelet’s Quetelet Index, a precursor to modern BMI calculations. BMI has become an essential metric in assessing weight status and its association with health outcomes.
How to Calculate BMI in SPSS
Calculating BMI in SPSS is essential for data analysis in medical research and healthcare. Here are 10 key aspects to consider:
- Data preparation
- Variable selection
- Formula application
- Output interpretation
- Categorization
- Statistical analysis
- Visualization techniques
- Reporting and communication
- Limitations and considerations
- Ethical implications
These aspects provide a comprehensive understanding of how to calculate BMI in SPSS, ensuring accurate and meaningful analysis. They encompass data management, statistical techniques, and ethical considerations, guiding researchers in effectively utilizing SPSS for BMI calculations.
Data preparation
Data preparation is a crucial step in the process of calculating BMI in SPSS. It involves ensuring that the data is accurate, complete, and in a format that SPSS can understand. There are several key aspects to consider when preparing data for BMI calculation:
- Data collection
Data collection involves gathering the necessary information to calculate BMI, such as height and weight. This data can be collected from surveys, medical records, or other sources. - Data cleaning
Data cleaning involves identifying and correcting errors in the data. This may include removing outliers, dealing with missing values, and checking for consistency. - Data transformation
Data transformation involves converting the data into a format that SPSS can understand. This may include converting units of measurement or creating new variables. - Data validation
Data validation involves checking the data to ensure that it is accurate and complete. This may involve using statistical techniques to identify errors or inconsistencies.
By carefully preparing the data, researchers can ensure that the BMI calculations are accurate and reliable. This is essential for conducting meaningful research and making informed decisions based on the results.
Variable selection
Variable selection is a critical aspect of calculating BMI in SPSS. It involves choosing the appropriate variables from the dataset to use in the BMI calculation. The variables selected should be relevant to the research question and should provide accurate and reliable results.
- Variable types
The type of variable selected will depend on the data available. Continuous variables, such as height and weight, are typically used for BMI calculations. Categorical variables, such as gender or age group, may also be used in some cases.
- Data source
The data source should be carefully considered when selecting variables. Data from surveys, medical records, or other sources may have different levels of accuracy and completeness.
- Measurement units
The units of measurement for the selected variables should be consistent. For example, height should be measured in meters or centimeters, and weight should be measured in kilograms or pounds.
- Data transformation
In some cases, data transformation may be necessary to prepare the variables for BMI calculation. For example, if height is measured in feet and inches, it may need to be converted to meters before it can be used in the BMI formula.
Careful consideration of variable selection is essential for obtaining accurate and reliable BMI calculations in SPSS. Researchers should carefully evaluate the available data and select variables that are relevant to the research question and that meet the requirements of the BMI formula.
Formula application
Formula application is a central aspect of calculating BMI in SPSS. It involves using the correct formula to calculate BMI based on the selected variables. The formula used is:
BMI = weight (kg) / height (m)^2
- Formula components
The formula consists of two components: weight and height. Weight should be measured in kilograms (kg), and height should be measured in meters (m) for accurate results.
- Data transformation
In some cases, data transformation may be necessary before applying the formula. For example, if height is measured in feet and inches, it should be converted to meters before using it in the formula.
- Rounding
The calculated BMI value may have several decimal places. Typically, BMI is rounded to one decimal place for reporting purposes.
- Interpretation
Once BMI is calculated, it can be interpreted using standard BMI categories. These categories range from underweight to obese, providing insights into an individual’s weight status.
Understanding and correctly applying the BMI formula is crucial for obtaining accurate and reliable results in SPSS. Researchers should carefully follow the formula and consider any necessary data transformations to ensure the validity of their BMI calculations.
Output interpretation
Output interpretation is a crucial component of calculating BMI in SPSS. The formula used to calculate BMI produces a numerical value, but it is essential to interpret this value correctly to derive meaningful insights. The interpretation involves comparing the calculated BMI to established BMI categories, which range from underweight to obese. Accurate interpretation of the output allows researchers to classify individuals based on their weight status and assess the potential health risks associated with it.
Real-life examples of output interpretation in the context of BMI calculation in SPSS include using the calculated BMI value to identify individuals at risk of developing weight-related health conditions, such as heart disease, stroke, or diabetes. Researchers can also use BMI output to monitor changes in weight status over time, evaluate the effectiveness of weight management interventions, and compare BMI distributions across different populations.
Understanding the practical applications of output interpretation is essential for utilizing BMI calculations effectively in SPSS. Interpreted BMI values provide valuable information for healthcare professionals, researchers, and individuals seeking to manage their weight and improve their overall health. By correctly interpreting BMI output, researchers can make informed decisions, develop targeted interventions, and contribute to a better understanding of weight-related health issues.
Categorization
Categorization plays a vital role in the process of calculating BMI in SPSS. It involves classifying individuals into different weight status categories based on their calculated BMI values. This categorization allows researchers to identify individuals at risk of developing weight-related health conditions and to compare BMI distributions across different populations.
- BMI Categories
The most commonly used BMI categories are underweight, normal weight, overweight, and obese. These categories are defined by specific BMI ranges, which vary depending on age and sex.
- Clinical Implications
BMI categories have important clinical implications. Individuals who are underweight or obese are at increased risk of developing a range of health problems, including heart disease, stroke, and diabetes.
- Population Monitoring
Categorization of BMI is also used for population monitoring. By tracking the distribution of BMI categories over time, researchers can identify trends in weight status and assess the effectiveness of public health interventions.
- Research Applications
BMI categorization is widely used in research studies investigating the relationship between weight status and various health outcomes. It allows researchers to compare the health risks associated with different BMI categories and to identify factors that contribute to weight-related health problems.
In summary, categorization is a crucial aspect of calculating BMI in SPSS. It enables researchers to classify individuals into different weight status categories, which have important clinical and public health implications. By understanding the principles of BMI categorization, researchers can effectively utilize SPSS to analyze BMI data and draw meaningful conclusions.
Statistical analysis
Statistical analysis plays a crucial role in “how to calculate BMI in SPSS.” It involves applying statistical techniques to BMI data to uncover patterns, draw inferences, and make informed decisions. Statistical analysis allows researchers to go beyond mere calculation and delve into the deeper insights hidden within BMI data.
As a critical component of “how to calculate BMI in SPSS,” statistical analysis enables researchers to:
- Identify trends and patterns in BMI distribution across populations or subgroups.
- Assess the relationship between BMI and other health indicators, such as blood pressure, cholesterol levels, and mortality rates.
- Evaluate the effectiveness of weight loss interventions by comparing BMI before and after the intervention.
By leveraging statistical analysis, researchers can gain a comprehensive understanding of BMI data, leading to more accurate conclusions and evidence-based recommendations.
For instance, statistical analysis has been instrumental in establishing the association between obesity (high BMI) and increased risk of chronic diseases like heart disease and diabetes. This understanding has shaped public health policies and guidelines aimed at promoting healthy weight management.
In summary, “how to calculate BMI in SPSS” is incomplete without statistical analysis. Statistical techniques empower researchers to uncover valuable insights from BMI data, contributing to a deeper understanding of weight status, its determinants, and its implications for health outcomes. This understanding lays the foundation for effective interventions and evidence-based decision-making in the field of weight management.
Visualization techniques
Visualization techniques play a significant role in “how to calculate BMI in SPSS” by enabling researchers to present and analyze BMI data in a visual format. These techniques help identify patterns, trends, and outliers, enhancing the understanding and communication of BMI-related insights.
- Charts and graphs
Charts and graphs are commonly used to visualize BMI data. Line charts can show trends over time, bar charts can compare BMI across different groups, and scatterplots can reveal relationships between BMI and other variables.
- Maps
Maps can be used to visualize the geographic distribution of BMI data. This can help identify areas with high or low BMI prevalence and target interventions accordingly.
- 3D modeling
3D modeling can create interactive representations of BMI data. This allows researchers to explore data from different perspectives and gain a more comprehensive understanding of the relationships between variables.
- Virtual reality (VR)
VR can be used to create immersive experiences that allow researchers to interact with BMI data in a virtual environment. This can enhance the understanding of complex relationships and facilitate data exploration.
Visualization techniques are essential for effectively communicating BMI-related findings to stakeholders, including researchers, policymakers, and the general public. By presenting data in a visual format, researchers can make complex information more accessible and actionable.
Reporting and communication
Reporting and communication are integral aspects of “how to calculate BMI in SPSS.” Effectively conveying the calculated BMI values and their implications is crucial for informing decision-making, shaping health policies, and promoting public health.
- Data presentation
BMI data can be presented in various formats, including tables, graphs, and charts. Choosing the appropriate format depends on the audience and the intended purpose of the communication.
- Interpretation and contextualization
The calculated BMI values should be interpreted and contextualized within the appropriate clinical and public health frameworks. This involves comparing the values to established BMI categories and discussing the implications for health risks and treatment options.
- Communication channels
The choice of communication channel depends on the target audience. Research findings can be disseminated through scientific journals, conference presentations, policy briefs, or social media platforms.
- Audience engagement
Effective communication involves engaging the audience and making the findings relevant to their needs. This may involve using clear and concise language, avoiding jargon, and providing practical recommendations.
Reporting and communication play a vital role in translating BMI calculation results into actionable insights. By presenting data effectively, interpreting it accurately, choosing appropriate communication channels, and engaging the audience, researchers can maximize the impact of their findings and contribute to improved health outcomes.
Limitations and considerations
In “how to calculate BMI in SPSS,” limitations and considerations play a crucial role in ensuring accurate and reliable results. Limitations refer to the inherent constraints of the BMI formula and the data used, while considerations involve factors that can affect the interpretation and application of BMI values.
One key limitation of BMI is that it does not take into account factors such as body composition, muscle mass, and body frame size. As a result, individuals with high muscle mass or a large frame may be misclassified as overweight or obese based on BMI alone. Additionally, BMI may not be an accurate indicator of health risks for certain populations, such as the elderly or pregnant women.
To address these limitations, researchers should consider using additional measures of body composition, such as waist circumference or body fat percentage. It is also important to interpret BMI values within the context of an individual’s overall health status and medical history. By understanding the limitations and considerations associated with BMI, researchers can make more informed decisions about its use and interpretation.
In practice, these considerations have significant implications for healthcare professionals, policymakers, and individuals seeking to manage their weight. For instance, relying solely on BMI to assess health risks may lead to inaccurate conclusions and inappropriate treatment recommendations. Therefore, it is essential to consider the limitations and use BMI in conjunction with other relevant health indicators.
Ethical implications
Ethical implications are inherent in “how to calculate BMI in SPSS” due to the potential misuse or misinterpretation of BMI data. Understanding these ethical considerations is crucial for responsible and ethical research and practice.
- Data privacy and confidentiality
BMI data can be sensitive and should be treated with confidentiality. Researchers must ensure that data is collected, stored, and analyzed securely to protect individuals’ privacy.
- Informed consent
Individuals should be fully informed about the purpose and implications of BMI calculation and provide their consent before participating in research or clinical settings.
- Stigma and discrimination
BMI is often associated with weight bias and stigma. Researchers and healthcare professionals should use BMI data responsibly and avoid stigmatizing individuals based on their BMI.
- Equity and access
BMI calculation should be accessible to all individuals, regardless of their background or circumstances. Researchers and policymakers should address disparities in access to BMI calculation and ensure equitable health outcomes.
These ethical implications underscore the importance of responsible and ethical practices in “how to calculate BMI in SPSS.” By adhering to these principles, researchers and healthcare professionals can contribute to a more just and equitable society.
Frequently Asked Questions (FAQs)
This FAQ section addresses common questions and clarifies key aspects related to “how to calculate BMI in SPSS.” These questions provide guidance on data preparation, formula application, interpretation, and ethical considerations.
Question 1: What data is required to calculate BMI in SPSS?
Answer: To calculate BMI in SPSS, you need two variables: weight in kilograms and height in meters.
Question 2: How do I handle missing data when calculating BMI?
Answer: Missing data can be imputed using various techniques, such as mean imputation or multiple imputation. The choice of imputation method depends on the nature of the missing data and the research question.
Question 3: Are there any limitations to using BMI as a measure of health?
Answer: While BMI is widely used, it has limitations. It does not account for factors such as body composition, muscle mass, or frame size. Therefore, it may not be an accurate indicator of health status for certain individuals.
Question 4: How can I ensure the ethical use of BMI data?
Answer: Ethical considerations include protecting data confidentiality, obtaining informed consent, and avoiding stigmatization based on BMI. Researchers should use BMI data responsibly and within the context of an individual’s overall health.
Question 5: What are some common pitfalls to avoid when calculating BMI in SPSS?
Answer: Common pitfalls include using incorrect measurement units, not considering data quality, and misinterpreting BMI categories. Careful data preparation and analysis are crucial to ensure accurate and meaningful results.
Question 6: How can I further explore the topic of BMI calculation in SPSS?
Answer: To delve deeper into BMI calculation in SPSS, you can refer to the SPSS documentation, online tutorials, or seek guidance from a statistician or data analyst.
These FAQs provide a foundation for understanding the essential aspects of BMI calculation in SPSS. They highlight potential pitfalls and ethical considerations to ensure responsible data analysis. The next section of the article will explore advanced techniques and applications of BMI calculation in SPSS.
Tips for Calculating BMI in SPSS
This section provides practical tips to help you accurately and effectively calculate BMI in SPSS. By following these tips, you can ensure the reliability and validity of your results.
Tip 1: Ensure accurate data entry. Verify that the weight and height values entered into SPSS are correct and consistent with the measurement units used.
Tip 2: Check for missing data. Identify and handle missing data appropriately using imputation techniques or excluding cases with missing values.
Tip 3: Apply the correct formula. Use the standard BMI formula (BMI = weight (kg) / height (m)^2) and ensure that the weight and height values are in the appropriate units.
Tip 4: Interpret BMI values correctly. Refer to established BMI categories and consider factors such as age, sex, and ethnicity when interpreting BMI values.
Tip 5: Consider limitations and ethical implications. Be aware of the limitations of BMI as a health indicator and handle BMI data ethically, respecting individuals’ privacy and avoiding stigmatization.
Tip 6: Use visualization techniques. Create graphs and charts to visualize BMI data, which can help identify patterns and trends.
Tip 7: Report results clearly and transparently. Present BMI results in a clear and informative manner, including relevant statistical measures and contextual information.
Following these tips will enhance the accuracy and reliability of your BMI calculations in SPSS, enabling you to draw meaningful conclusions from your data.
The next section of the article will delve into advanced techniques for analyzing BMI data in SPSS, such as statistical modeling and data mining techniques.
Conclusion
This article has provided a comprehensive guide on “how to calculate BMI in SPSS,” covering essential aspects such as data preparation, formula application, output interpretation, and ethical considerations. Understanding these concepts is crucial for accurate and meaningful BMI calculations in SPSS.
Key points to remember include:
- Careful data preparation and variable selection are essential for reliable BMI calculations.
- Correct application of the BMI formula and interpretation of results are vital to avoid misclassification.
- Ethical considerations, such as data privacy and avoiding stigmatization, should be prioritized in BMI data analysis.
The insights gained from this article empower researchers and practitioners to effectively calculate and utilize BMI data in SPSS. By adhering to these principles, researchers can contribute to a deeper understanding of weight status, its determinants, and its implications for health outcomes.
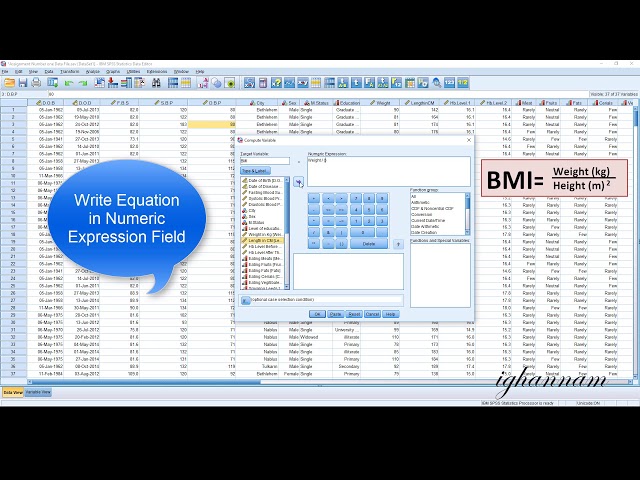