BMI, or body mass index, is a measure of body fat based on height and weight. Simply put, it helps determine whether someone is underweight, normal, overweight, or obese.
Calculating BMI is crucial for understanding one’s health status and risk of chronic diseases. It plays a vital role in clinical settings, nutritional assessments, and epidemiological studies. The concept was first introduced in the 19th century, when Belgian statistician Adolphe Quetelet developed the Quetelet Index, which later evolved into BMI.
In this article, we will provide a step-by-step guide on how to calculate BMI using SPSS, exploring the underlying formula, input variables, and interpretation of results.
How to Calculate BMI on SPSS
Understanding the essential aspects of calculating BMI on SPSS is crucial for accurate interpretation and application in health-related research and practice.
- Formula
- Input Variables
- Interpretation
- Categorization
- Health Implications
- Limitations
- Statistical Significance
- SPSS Syntax
- Example Analysis
- Reporting Results
These aspects provide a comprehensive framework for understanding the calculation, interpretation, and application of BMI using SPSS. They encompass the technical foundations, statistical considerations, and practical implications of BMI analysis.
Formula
The formula for calculating BMI is crucial in the context of “how to calculate BMI on SPSS” because it serves as the mathematical foundation for the calculation process. Without the formula, it would not be possible to determine BMI values based on the input data.
The BMI formula, BMI = weight (kg) / height (m2), is a critical component of “how to calculate BMI on SPSS” as it establishes the precise mathematical relationship between weight and height. The formula ensures that BMI values are calculated consistently and accurately, allowing for meaningful comparisons and interpretations.
In practice, “how to calculate BMI on SPSS” utilizes the formula to convert raw weight and height data into BMI values. These values can then be categorized into different weight status groups (e.g., underweight, normal weight, overweight, obese) based on established cut-off points. This categorization helps healthcare professionals assess a patient’s weight status and identify potential health risks.
Understanding the formula provides a deeper comprehension of the calculation process and its implications. It allows researchers and practitioners to interpret BMI values correctly and make informed decisions regarding weight management and health interventions.
Input Variables
Input variables play a pivotal role in “how to calculate BMI on SPSS” because they constitute the raw data upon which BMI values are derived. The accuracy and validity of BMI calculations heavily depend on the quality and completeness of the input variables.
In the context of “how to calculate BMI on SPSS,” the primary input variables are weight and height, which must be entered accurately into the SPSS software. Weight is typically measured in kilograms (kg), while height is measured in meters (m) or centimeters (cm) and converted to meters within SPSS. These variables serve as the foundation for BMI calculations, and any errors or omissions in their input can significantly affect the calculated BMI values.
Understanding the relationship between input variables and “how to calculate BMI on SPSS” is crucial for researchers and practitioners. It underscores the importance of accurate data entry and data management practices to ensure reliable BMI calculations. Moreover, it highlights the need for careful consideration of the units of measurement used for weight and height to avoid errors and ensure consistency in BMI calculations across different studies and settings.
Interpretation
Interpretation is paramount to “how to calculate BMI on SPSS” as it allows researchers and healthcare professionals to make meaningful sense of the calculated BMI values. Without interpretation, the numerical BMI values remain mere numbers, devoid of clinical significance or actionable insights.
BMI interpretation involves comparing the calculated BMI value against established cut-off points or reference values. These cut-off points categorize individuals into different weight status groups, such as underweight, normal weight, overweight, or obese. This categorization provides valuable information about an individual’s weight status and potential health risks associated with it.
For instance, in the context of “how to calculate BMI on SPSS,” a BMI value below 18.5 kg/m2 may be interpreted as underweight, indicating a potential risk of malnutrition or eating disorders. Conversely, a BMI value of 30 kg/m2 or higher may be interpreted as obese, suggesting an increased risk of chronic diseases such as heart disease, stroke, and type 2 diabetes.
Understanding the interpretation of BMI values derived from “how to calculate BMI on SPSS” is essential for healthcare professionals to make informed clinical decisions. It enables them to identify individuals at risk of weight-related health problems and develop appropriate interventions to promote healthy weight management.
Categorization
Within the context of “how to calculate BMI on SPSS,” categorization plays an integral role by organizing and interpreting the calculated BMI values. This process involves classifying individuals into distinct weight status groups based on established cut-off points or reference values, such as underweight, normal weight, overweight, and obese.
Categorization serves as a critical component of “how to calculate BMI on SPSS” as it provides a meaningful interpretation of the numerical BMI values, allowing healthcare professionals to assess an individual’s weight status and potential health risks. The categorization process transforms the raw BMI values into actionable information, guiding clinical decision-making and facilitating targeted interventions.
Real-life examples of categorization within “how to calculate BMI on SPSS” include the identification of individuals who are underweight (BMI < 18.5 kg/m2), normal weight (18.5 BMI < 25 kg/m2), overweight (25 BMI < 30 kg/m2), or obese (BMI 30 kg/m2). These categorizations help healthcare professionals prioritize care, develop appropriate weight management strategies, and provide targeted health education and counseling.
Understanding the connection between “Categorization” and “how to calculate BMI on SPSS” enhances the practical application of BMI analysis in various settings. It enables researchers and practitioners to interpret and communicate BMI values effectively, facilitating evidence-based decision-making and improving patient care.
Health Implications
Understanding the health implications associated with BMI is critical within the context of “how to calculate BMI on SPSS.” BMI serves as an indicator of an individual’s weight status, providing insights into potential health risks and informing clinical decision-making.
- Disease Risk Assessment
BMI is a crucial factor in assessing the risk of developing chronic diseases such as cardiovascular disease, stroke, type 2 diabetes, and certain types of cancer. Higher BMI values are associated with an increased risk of these conditions.
- Metabolic Abnormalities
BMI is closely linked to metabolic abnormalities, including dyslipidemia (abnormal cholesterol levels), insulin resistance, and impaired glucose tolerance. These abnormalities can contribute to the development of cardiovascular disease and type 2 diabetes.
Overall, the health implications of BMI underscore the importance of maintaining a healthy weight. By calculating BMI using SPSS, healthcare professionals can identify individuals at risk of developing weight-related health problems and implement appropriate interventions to promote healthy weight management and improve overall health outcomes.
Limitations
While calculating BMI using SPSS offers valuable insights, it is essential to acknowledge its limitations to ensure accurate interpretation and appropriate application in healthcare settings.
- Accuracy of Input Data
The accuracy of BMI calculations heavily relies on the accuracy of the input data, particularly weight and height. Errors in data entry or missing values can significantly affect the calculated BMI values, potentially leading to misclassification of weight status.
- Body Composition
BMI does not account for individual differences in body composition. For instance, individuals with a high muscle mass may have a higher BMI despite having a healthy body fat percentage. This limitation can result in the misclassification of certain individuals, such as athletes or bodybuilders.
- Age and Ethnicity
BMI cut-off points for defining weight status categories may not be universally applicable across different age groups and ethnicities. Age- and ethnicity-specific BMI references may be more appropriate in certain populations to account for variations in body composition and health risks.
- Overall Health Status
BMI alone does not provide a complete picture of an individual’s overall health status. Other factors, such as medical conditions, medications, and lifestyle behaviors, can influence weight and health outcomes. Relying solely on BMI may overlook these important considerations.
Understanding the limitations of BMI calculations using SPSS is crucial for healthcare professionals to interpret results appropriately and make informed clinical decisions. Considering these limitations alongside other health indicators ensures a more comprehensive assessment of an individual’s weight status and overall health.
Statistical Significance
Statistical significance plays a crucial role in “how to calculate BMI on SPSS” by providing a quantitative measure of the likelihood that observed differences in BMI values are due to actual underlying factors rather than chance.
- Hypothesis Testing
Statistical significance is used in hypothesis testing to determine whether the observed difference between two groups is statistically significant, meaning that it is unlikely to have occurred by chance alone.
- p-Values
The p-value is a numerical measure of statistical significance, representing the probability of obtaining a result as extreme or more extreme than the one observed, assuming the null hypothesis is true.
- Confidence Intervals
Confidence intervals provide a range of values within which the true population parameter is likely to fall, based on the sample data and the level of statistical significance.
- Effect Size
Effect size measures the magnitude of the observed difference between groups, regardless of statistical significance, and provides insights into the practical importance of the findings.
Understanding statistical significance in the context of “how to calculate BMI on SPSS” enables researchers to draw meaningful conclusions from their data, make informed decisions, and communicate their findings with clarity and precision.
SPSS Syntax
SPSS Syntax is a powerful tool within “how to calculate BMI on SPSS” as it enables researchers to automate the computation of BMI values, manage and transform data, and perform statistical analyses in a standardized manner.
- Syntax Commands
SPSS Syntax consists of specific commands that instruct the software to perform various tasks, such as reading data, calculating BMI, and generating statistical outputs.
- Batch Processing
SPSS Syntax allows for batch processing, where multiple commands can be executed sequentially, reducing manual effort and increasing efficiency in handling large datasets.
- Reproducibility
SPSS Syntax promotes reproducibility by providing a detailed record of the analytical procedures performed, enabling others to replicate the analysis and verify the results.
- Customization
SPSS Syntax offers customization options, allowing researchers to tailor the analysis to their specific research questions and data requirements.
Overall, SPSS Syntax plays a vital role in “how to calculate BMI on SPSS” by automating and standardizing the analytical process, enhancing efficiency, reproducibility, and customization, ultimately contributing to the accuracy and reliability of BMI calculations and statistical inferences.
Example Analysis
Example analysis in the context of “how to calculate BMI on SPSS” plays a pivotal role in understanding the practical application and interpretation of BMI calculations. It involves examining real-world scenarios and data to illustrate the computation and interpretation of BMI values.
- Components of BMI Calculation
Example analysis demonstrates the step-by-step process of calculating BMI, including data input, formula application, and result interpretation.
- Real-Life Applications
Examples illustrate how BMI is used in clinical settings, epidemiological studies, and nutritional assessments to assess weight status and health risks.
- Interpretation of Results
Example analysis helps researchers and healthcare professionals understand how to interpret BMI values in relation to established cut-off points and weight status categories.
- Implications for Health
Examples highlight the implications of BMI for overall health and disease risk, emphasizing the importance of maintaining a healthy weight.
Overall, example analysis is a valuable tool in “how to calculate BMI on SPSS” as it provides practical insights into the calculation process, interpretation of results, and real-world applications, ultimately promoting accurate and meaningful BMI analysis for health assessment and intervention.
Reporting Results
Reporting results is a critical component of “how to calculate BMI on SPSS” as it allows researchers and practitioners to effectively communicate their findings and contribute to the broader understanding of weight-related health issues. Accurate and transparent reporting ensures the validity and reliability of BMI calculations and statistical analyses.
Clear and concise reporting involves presenting the calculated BMI values in an organized and meaningful manner. This includes providing descriptive statistics, such as mean, median, and standard deviation, to summarize the distribution of BMI values within the sample.
Furthermore, researchers may choose to present BMI values in graphical formats, such as histograms or scatterplots, to visually illustrate the distribution of data and identify potential patterns or outliers. Additionally, reporting the results in the context of established cut-off points or reference values helps readers interpret the weight status of the sample and identify individuals who may be at risk of weight-related health problems.
The practical applications of understanding the connection between “Reporting Results” and “how to calculate BMI on SPSS” are vast. It enables researchers to disseminate their findings to the scientific community, policymakers, and the public, contributing to evidence-based decision-making and health promotion initiatives.
Frequently Asked Questions
This section addresses common inquiries and clarifications regarding “how to calculate BMI on SPSS”.
Question 1: What is the formula used to calculate BMI on SPSS?
Answer: The formula for BMI calculation in SPSS is BMI = weight (kg) / height (m2).
Question 2: How do I enter weight and height data into SPSS for BMI calculation?
Answer: Create two separate variables in SPSS, one for weight in kilograms and the other for height in meters. Ensure that the data is entered accurately to obtain correct BMI values.
Question 3: What are the established cut-off points for BMI categories?
Answer: The World Health Organization (WHO) defines the BMI categories as follows: underweight (<18.5 kg/m2), normal weight (18.5 – 24.9 kg/m2), overweight (25 – 29.9 kg/m2), and obese (30 kg/m2).
Question 4: How can I interpret the calculated BMI values?
Answer: BMI values should be interpreted in the context of established cut-off points. Individuals with a BMI below 18.5 kg/m2 are considered underweight, while those with a BMI of 30 kg/m2 or higher are considered obese.
Question 5: What are the limitations of using BMI as a measure of health?
Answer: BMI does not account for individual differences in body composition, such as muscle mass and body fat distribution. It may not accurately reflect health risks for certain individuals, such as athletes or the elderly.
Question 6: How can I ensure the accuracy of BMI calculations using SPSS?
Answer: Check the accuracy of the entered weight and height data. Use appropriate data types (numeric for weight and height) and variable formats (kg for weight and m for height) to avoid errors.
These FAQs provide essential insights into the calculation, interpretation, and limitations of BMI using SPSS. Understanding these aspects is crucial for accurate and meaningful BMI analysis in research and practice.
The next section will delve into advanced topics related to BMI calculation on SPSS, including syntax commands, customization options, and statistical considerations.
Tips for Calculating BMI on SPSS
This section provides practical tips to enhance the accuracy, efficiency, and interpretation of BMI calculations using SPSS.
Tip 1: Ensure Data Accuracy
Verify the accuracy of entered weight and height data to obtain reliable BMI values.
Tip 2: Use Appropriate Data Types
Specify the data type as numeric for weight and height variables to prevent errors during calculation.
Tip 3: Set Correct Variable Formats
Define the variable format as kilograms (kg) for weight and meters (m) for height to match the BMI formula.
Tip 4: Utilize SPSS Syntax Commands
Automate BMI calculations using SPSS syntax commands, reducing manual effort and minimizing errors.
Tip 5: Check for Outliers
Examine the calculated BMI values for extreme outliers that may indicate data entry errors or exceptional cases.
Tip 6: Interpret Results in Context
Relate BMI values to established cut-off points to assess weight status and identify individuals at risk of health concerns.
Tip 7: Consider Body Composition Limitations
Acknowledge that BMI does not account for variations in body composition, and supplement it with other health indicators as appropriate.
Key Takeaways and Benefits:
Following these tips enhances the accuracy and reliability of BMI calculations on SPSS, leading to more informed decision-making in healthcare and research.
As discussed, calculating BMI on SPSS involves crucial considerations and potential limitations. To further explore these aspects, the next section will delve into advanced topics, including statistical significance, reporting results, and addressing common challenges.
Conclusion
This comprehensive exploration of “how to calculate BMI on SPSS” has elucidated the formula, input variables, interpretation, and limitations of BMI calculations within the SPSS software. By understanding these aspects, researchers and healthcare professionals can accurately assess weight status and identify individuals at risk of weight-related health problems.
Key takeaways include the importance of precise data entry, appropriate variable formats, and consideration of BMI’s limitations, such as its inability to account for body composition variations. Additionally, utilizing SPSS syntax commands can enhance efficiency and minimize errors in BMI calculations.
Calculating BMI on SPSS remains a valuable tool for assessing weight status in research and clinical settings. As the prevalence of weight-related health issues continues to rise, accurate and standardized BMI calculations are crucial for developing effective interventions and promoting healthy weight management.
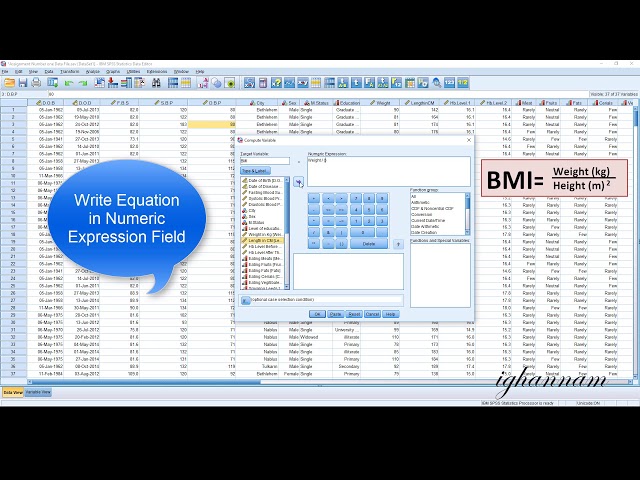